https://arxiv.org/abs/2005.07025
Converting Anyone's Emotion: Towards Speaker-Independent Emotional Voice Conversion
Emotional voice conversion aims to convert the emotion of speech from one state to another while preserving the linguistic content and speaker identity. The prior studies on emotional voice conversion are mostly carried out under the assumption that emotio
arxiv.org
해당 논문을 보고 작성했습니다.
Abstract
emotional voice conversion은 linguistic content와 speaker identity를 유지한 채로 speech의 감정을 변환하는 것을 목표로 합니다. 대부분의 이전 emotional voice conversion 연구는 emotion이 speaker-dependent 하다는 가정 하에 진행되었습니다. 저자들은 spoken language에서 감정 표현은 화자를 초월한 공통적인 code가 있다고 가정하며, emotion state 사이 speaker-independent mapping이 가능하다고 합니다. 이 논문에서, 저자들은 parallel data 없이도 누구나의 emotion을 변환할 수 있는 speaker-independent emotional voice conversion framework를 제안합니다. 저자들은 VAW-GAN based encoder-decoder structure를 제안하며, 이를 통해 spectrum and prosody mapping을 학습합니다. 시간 의존성을 modeling 하기 위해, 저자들은 continuous wavelet transform (CWT)을 사용하여 prosody conversion을 수행하였습니다. 또한 저자들은 F0를 추가적인 input으로 decoder에 제공하여 emotion conversion performance를 향상시켰습니다.
Introduction
Spectral mapping은 conventional voice conversion에서 main focus를 받았지만, prosody mapping은 동일한 수준의 attention을 받진 못했습니다. 감정은 본질적으로 supra-segmental이고 hierarchical 한 특성을 가지고 있으며, spectrum과 prosody에도 영향을 미칩니다. 그러므로 frame 별로 spectral feature만 변환하는 것은 emotional voice conversion을 수행하기 불충분합니다.
prosody conversion을 위한 statistical modelling은 성공을 거두었습니다. classificiation regression tree를 이용하여 pitch contour을 계층적 구조로 분해한 후 변환하는 연구가 있었습니다. 또 다른 방식은 source and target dictionary를 생성한 후, sparse mapping을 추정하는 NMF 기반 기법을 활용하는 연구가 있습니다. 뿐만 아니라 HMM, GMM, F0 segment selection method를 결합한 emotional voice conversion model도 등장하였습니다.
위 방식들은 parallel training data가 필요합니다. 이러한 필요성을 제거하기 위해, autoencoder and cycle-consistent generative adversarial network (Cycle-GAN) based emotional voice conversion framework가 등장하였습니다. 하지만 이 framework는 특정 화자에 맞춰 design 되며, speaker-dependent framework라 부릅니다.
감정 표현과 감정 인지는 화자의 성격, 언어, 문화 등에 따라 개인마다 variation이 존재합니다. 동시에 화자의 identity와 background와 관련 없이 공통된 cue를 공유합니다. emotion recognition 분야에서는 speaker-independent emotion recognition이 speaker dependent recognition보다 더 robust, stable, generalization 하다는 것을 입증하였습니다. 하지만 speaker-independent emotional voice conversion에 대한 연구가 거의 없습니다. 대부분의 model은 multi-speaker model을 다루고 있습니다.
CycleGAN은 parallel training data 없이도 voice conversion을 수행할 수 있는 효과적인 solution이긴 하지만, pair-wise conversion에서 더 안정적입니다. variational autoencoder Wasserstein generative adversarial network (VAW-GAN)과 같은 encoder-decoder 구조는 emotion-independent representation을 학습하는 데 더욱 안정적입니다. 이 논문의 contribution은 다음과 같습니다. 1) speaker-independent voice conversion에 대한 연구를 진행합니다. 2) VAW-GAN 구조를 제안하며, parallel training data 없이도 학습이 가능합니다. 3) prosody modelling에 대한 연구를 진행하였으며, emotion-independent encoder training을 위한 F0 conditioning을 제안합니다.
Speaker-Independent Perspective on Emotion
speech는 단순한 단어 조합이 아닙니다. 화자의 감정을 담고 있습니다. 감정은 화자의 의도, 분위기, 기질을 반영하며, 대화를 하고 의견을 표현하는 데 있어 중요한 역할을 합니다. 감정은 spectrum과 prosody에 관련된 여러 signal 특성들을 포함하는 복합적인 요소이며, 분리하거나 합성하기 어렵습니다.
이전 연구들은 인간 문화 전반에 걸친 universal principle을 통해 basic emotion을 표현하고 인지할 수 있다는 것을 발견하였습니다. 일반적으로 speech에 존재하는 감정 상태는 glottal source와 vocal tract 전반에 걸쳐 speech를 생성하는 mechanism에 영향을 준다고 알고 있습니다. 이러한 연구들은 large multi-speaker emotional corpus, emotion feature extraction and classifier를 이용하여 seen and unseen speaker에 대한 speaker-independent emotion representation이 가능함을 시사하였습니다.
speaker-independent emotion element가 존재하는지 검증하기 위해, 저자들은 speaker-dependent EVC를 위해 design 된 CycleGAN-based emotional voice conversion framework을 이용해 사전 실험을 진행했습니다. 이 실험에서, spectrum and prosody (CWT-based F0 feature)을 mapping 하는 2가지 conversion pipeline을 학습시켰습니다. 이 network를 특정 화자로 학습하고 seen and unseen speaker에 대해 test를 진행하였습니다.
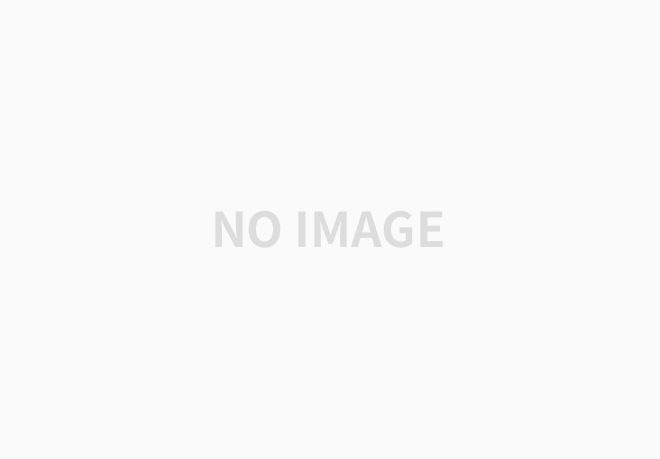
실험 결과는 위와 같습니다. Mel-cepstral distortion (MCD)와 log-spectral distortion (LSD)를 이용해 spectrum conversion 성능을 확인하였으며, Pearson correlation coefficient (PCC)와 F0 contour의 root mean square error (RMSE)으로 prosody conversion에 대한 평가를 진행하였습니다. zero effort는 source speech에 어떠한 변환을 수행하지 않고 target emotion과 비교한 결과입니다.
speaker-dependent CycleGAN system이 unseen speaker에서도 어느 정도 잘 동작하는 것을 볼 수 있습니다. unseen speaker에 대한 실험 결과는 확실히 zero effort보다 성능이 뛰어난 모습을 보입니다. 이를 통해 저자들은 어떤 화자든 감정을 변환할 수 있는 speaker-independent emotional voice conversion을 수행하는 framework를 제안합니다.
Speaker-Independent EVC
VAW-GAN과 같은 encoder-decoder 구조는 encoder를 이용해 emotion-independent representation을 학습할 수 있게 만들어 줍니다. CycleGAN 대신, 저자들은 speaker-independent emotional voice conversion framework를 formulate 하기 위해 VAW-GAN의 encoder-decoder structure를 사용하였습니다.
WORLD vocoder를 이용해 spectral (SP)와 F0 feature를 추출합니다. F0는 segmental information을 포함하기 때문에, logarithm gaussian (LG)-based linear transformation을 이용해 F0 contour를 변환하는 것은 충분하지 않습니다. 저자들은 micro-prosody level부터 utterance level까지 prosody를 분석하기 위해, CWT decomposition을 이용해 F0를 분해합니다. 저자들은 F0 contour에 존재하는 speaker-dependent and independent component를 다르게 처리해야 한다고 생각합니다. F0의 CWT decomposition은 encoder가 두 speaker 사이 speaker-independent emotion pattern을 학습할 수 있게 만들어줍니다. CWT가 F0에 있는 불연속성에 sensitive 하기 때문에, 전처리과정이 필요합니다. 과정은 다음과 같습니다. 1) unvoiced region에 linear interpolation을 수행합니다. 2) linear F0를 logarithmic scale로 변환합니다. 3) 구해진 F0를 zero mean and unit variance로 normalize 합니다. 이 과정 이후 CWT decomposition을 수행합니다.
Training
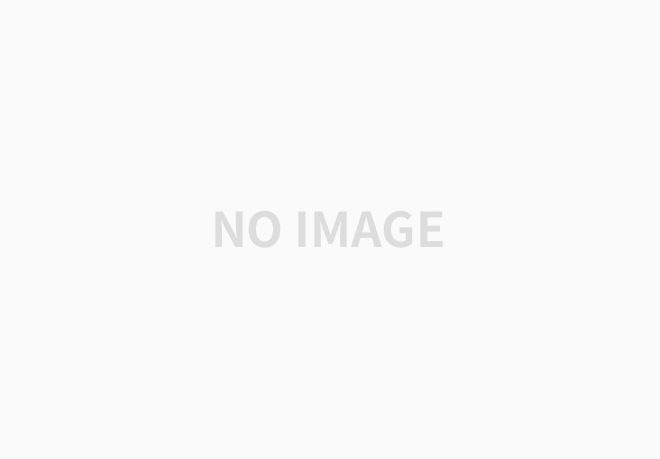
proposed VAW-GAN과 학습 과정은 위와 같습니다. spectrum을 학습하는 것과 prosody를 학습하는 것을 분리하는 것이 동시에 학습하는 것보다 더 좋은 성능을 달성한다는 연구가 존재합니다. 그러므로, 저자들도 두 network를 분리하여 학습하는 것을 제안합니다. 1) spectrum conversion의 경우 F0를 condition으로 사용하는 VAW-GAN model을 사용하며, 이를 VAW-GAN for Spectrum이라 부릅니다. 2) prosody conversion에 대한 VAW-GAN model은 VAW-GAN for Prosody 라 부릅니다. 각 network는 encoder, generator/decoder, discriminator로 구성됩니다.
VAW-GAN for Spectrum과 VAW-GAN for Prosody를 학습할 때, encoder는 여러 화자의 다양한 감정에서 추출한 input frame을 사용합니다. encoder는 다양한 화자를 통해 emotion-independent pattern을 학습하고 input feature를 latnet code z로 변환합니다. 이 latent code z는 emotion-independent 하고 speaker identity와 phonetic content만 포함하고 있습니다. 저자들은 one-hot vector를 이용해 emotion ID를 나타내며 이를 이용해 generator와 decoder에게 감정 정보를 제공합니다. VAW-GAN for Spectrum training에 있는 spectral feature는 F0에 매우 의존적이며 prosodic information을 많이 포함하고 있기 때문에, 저자들은 F0를 decoder의 추가적인 condition으로 사용하여 prosody로부터 spectral feature를 분리합니다.
그다음 adversarial learning으로 spectrum and prosody generative model을 학습시킵니다. 이를 통해 emotion ID로 정의된 target emotion을 나타내는 high-quality converted speech를 달성하게 됩니다.
Run-time Conversion
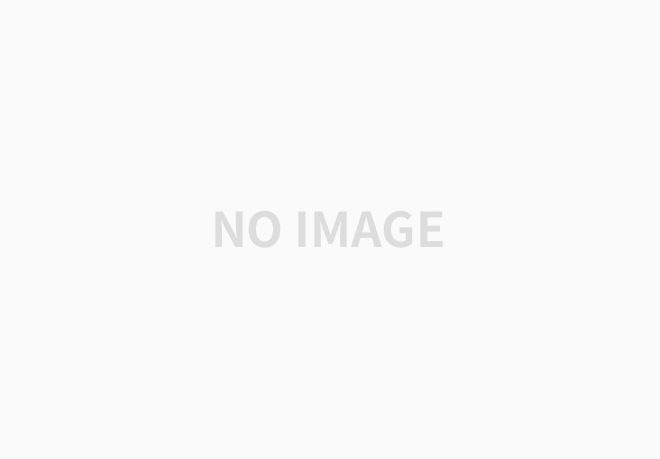
run-time conversion phase는 위와 같습니다. training phase와 유사하게, prosody conversion에서 CWT을 이용해 F0를 다양한 time scale의 coefficient로 분해하고 prosody encoder에 들어가 변환된 F0를 생성하게 됩니다. 이때 변환된 F0는 지정된 emotion ID를 반영합니다. spectrum conversion의 경우, generator/decoder는 converted CWT-based F0 coefficient와 emotion ID를 condition으로 합니다. 그 다음 trained VAW-GAN for Spectrum을 통해 converted spectral feature를 얻습니다. 최종적으로 WORLD vocoder를 이용해 converted emotional speech를 합성합니다. aperiodicity (AP)는 source speech에서 직접 복사하여 사용합니다.
Effect of F0 Conditioning
spectrum and prosody conversion의 encoder는 여러 화자의 다양한 감정으로부터 input feature를 추출하여 학습에 사용하며, emotion-independent latent code z를 생성합니다. decoder는 target emotion ID를 condition으로 하여 speech를 생성합니다. spectral feature가 F0 feature에 상당히 dependent 하면서 prosodic information을 담고 있기 때문에, one-hot emotion ID만 이용하여 emotion-independent encoder를 학습하는 건 불충분합니다. 그러므로 저자들은 F0를 generator의 추가적인 input으로 사용하였으며, 이를 통해 encoder가 emotion-independent representation만 학습하도록 만들었습니다. F0를 condition으로 사용하면 baseline에 비해 눈에 띄는 성능 향상을 보였습니다.
Comparison wiht Related Work
저자들의 EVC framework는 1) parallel training data가 필요 없으며, 2) alignment technique을 사용하지 않고, 3) 어떠한 speaker embedding도 필요하지 않고, 4) speech recognizer와 같은 외부 module을 사용하지 않습니다. 이는 F0 conditioning과 관련 있는 conditional VAE 기반 VC framework와 비슷한 motivation을 공유하지만, 많이 다릅니다. 예를 들어 speaker identity conversion에 focus 하지만, 기존 연구는 speaker identity conversion에 집중하였습니다. 그리고 저자들은 latent code z이 emotion-independent 하도록 residual prosodic information을 제거하는 F0 conditioning mechanism을 사용합니다. 이를 통해 emotion perspective는 없는 speaker-independent latent code를 생성합니다. 그리고 converted CWT-based F0 feature를 generator/decoder의 condition으로 사용하여 run-time을 구현했습니다. 이는 이전 연구에는 등장하지 않은 방식입니다.
Experiments
large emotional multi-speaker voice conversion dataset이 publicly available 하지 않기 때문에, 저자들은 3가지 emotional speech corpora를 사용하였습니다. English emotional speech corpus, EmoV-DB, JL-Corpus가 그 3가지입니다. 저자들은 처음 2개 dataset에서 3명 여성 화자를 선택해 network를 학습시켰으며, emotion conversion은 해당 3명 speaker와 JL-Corpus에서 random 하게 선택한 2명의 여성 화자에 대해 수행하였습니다. JL-Corpus에서 선택한 2명의 화자가 unseen speaker입니다. 학습에서 사용했던 화자는 seen speaker입니다.
저자들은 3가지 dataset에 공통된 2가지 감정인 neutral, angry에 대한 실험을 진행하였습니다. 모든 실험은 neutral에서 angry로 변환하도록 진행되었습니다.
Objective Evaluation

spectrum and prosody conversion의 performance를 평가하기 위해 objective evaluation을 진행했습니다. MCD와 LSD를 사용해 spectrum conversion evaluation을 진행하였으며, PCC를 이용해 prosody conversion evaluation을 진행하였습니다. 저자들이 제안한 Figure 1의 framework를 CWT-C-VAWGAN으로 표기합니다. C-VAWGAN은 CWT decomposition을 수행하지 않고 LG-based linear transformation으로 F0를 변환하고 condition으로 사용하는 VAW-GAN을 의미합니다. 실험 결과는 위와 같습니다.
저자들이 제안한 CWT-C-VAWGAN framework가 baseline보다 더 뛰어난 성능을 보여주는 것을 알 수 있습니다. CWT-based converted F0 conditioning이 LG-based F0 feature보다 더 효과적임을 알 수 있습니다. CWT-C-VAWGAN은 unseen에 대해서도 seen과 비교할 수 있을 정도의 성능을 보여줍니다. 이 결과를 통해 speaker independent EVC이 가능함을 입증하였습니다.
Subjective Evaluation
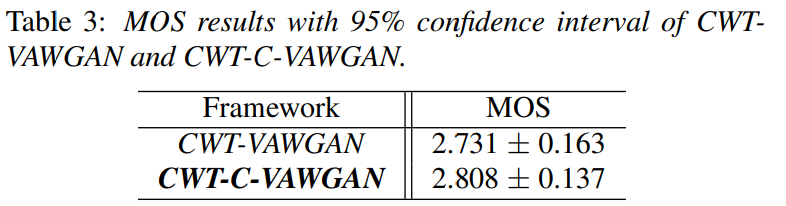
저자들은 MOS로 subjective evaluation을 진행하였으며, 저자들이 제안한 CWT-C-VAWGAN이 더 뛰어난 모습을 보였습니다.
Discussion
저자들이 제안한 framework는 spectrum conversion 및 prosody conversion 모두에서 speaker-independent emotional expression pattern을 학습합니다. 저자들이 제안한 CWT-based F0 conditioning이 spectrum conversion 성능을 향상시킵니다. 그리고 anyone's emotion converting이 가능합니다. 저자들이 알기론, 이 논문이 speaker-independent emotion conversion을 수행하는 첫 연구입니다.
Conclusion
저자들은 parallel data를 사용하지 않고도 anyone's emotion을 변환할 수 있는 speaker-independent EVC framework를 제안합니다. VAW-GAN 기반으로 spectrum and prosody conversion을 수행합니다. 저자들은 CWT으로 F0를 modeling 하며 성능을 향상시켰습니다. 실험을 통해 speaker-independent EVC가 seen and unseen speaker에 대해서 뛰어난 성능을 보인다는 것을 입증하였습니다.