https://arxiv.org/abs/2107.03748
Expressive Voice Conversion: A Joint Framework for Speaker Identity and Emotional Style Transfer
Traditional voice conversion(VC) has been focused on speaker identity conversion for speech with a neutral expression. We note that emotional expression plays an essential role in daily communication, and the emotional style of speech can be speaker-depend
arxiv.org
해당 논문을 보고 작성했습니다.
Abstract
Traditional voice conversion (VC)는 neutral expression speech의 speaker identity를 변경하는 것에 초점을 둡니다. emotional expression은 일상생활 의사소통에서 중요한 역할을 하며, speech의 감정 표현은 speaker-dependent 할 수 있습니다. 이 논문에서는 speaker identity와 speaker-dependent emotional style을 동시에 변환하는 기술을 제안하며, expressive voice conversion이라 불립니다. 저자들은 여러 화자 간의 many-to-many mapping을 학습하는 StarGAN-based framework를 제안하며, parallel data 없이 speaker-dependent emotional style을 고려하도록 design 하였습니다. 이를 위해 pre-trained speech emotion recognition (SER) model에서 emotional style encoding을 얻어 generator의 condition으로 사용합니다.
Introduction
이 논문에서는 새로운 research topic인 Expressive Voice Conversion을 제안하며, speaker identity와 emotional style을 동시에 변환하는 solution을 제안합니다. human speech는 expressive 하고 emotive 한 특성이 있다고 알려져 있으며, 사람들은 행복, 슬픔과 같은 다양한 감정 상태를 표현합니다. 다양한 연구들은 emotional speech style이 speaker dependent element를 포함하고 있다는 것을 발견하였습니다.
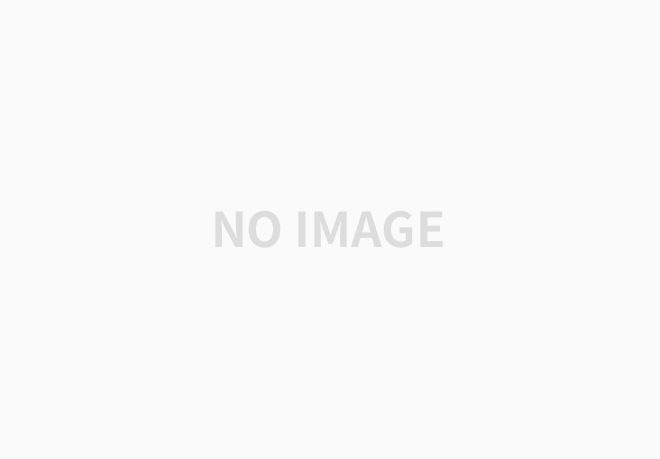
expressive voice conversion에서는 speaker identity와 emotional style 모두 다룹니다. 일반적으로 emotional speech style은 speaker identity보다 modeling하기 더 어렵고 복잡합니다. emotional speech style은 어휘 강세, 발화 행위와 연결된 보편적인 pattern인 speaker-independent emotional style과 개인적인 특성 & 배경 요소에 영향을 받는 speaker-dependent emotion style로 표현할 수 있다고 알려져 있습니다. speaker-independent element를 보존한 채로 speaker-dependent emotional style만 변경하는 것은 어렵기 때문에, expressive voice conversion이 challenging 한 task입니다.
expressive voice conversion은 emotional voice conversion과 유사하지만 많이 다릅니다. 예를 들어 emotional voice conversion은 speaker identity를 유지한 채로 emotional state만 변경하는 것을 목표로 합니다. emotional voice conversion에서는 generated speech와 target speech 사이의 emotional state similarity (e.g. happy, sad, angry)가 주요 해결 사항입니다. 하지만 expressive voice conversion에서는 감정 상태를 변경하지 않고, speaker identity와 emotional style 같은 speaker-dependent characteristic을 변환하는 것이 목표입니다.
일부 과거 연구에서는 Expressive voice conversion을 emotional voice conversion과 동일한 개념으로 간주한 사례가 있습니다. 이러한 혼동을 피하기 위해, 저자들은 expressive voice conversion을 'input speech를 target speaker의 speaker identity 및 emotional style로 변환하는 것' 으로 정의합니다. 저자들은 parallel data 없이도 conversion을 수행할 수 있는 expressive voice conversion framework를 제안합니다.
저자들이 제안한 framework는 2가지 학습 stage로 구성됩니다. 1) acoustic feature를 통해 emotional style feature와 같은 emotion-related information을 학습하도록 SER network를 학습시키는 과정인 emotional style descriptor training, 2) speaker label과 emotional style feature 둘 다 generator의 condition으로 사용하여 학습하는 과정인 Star-GAN training 이 있습니다. 저자들은 emotional style feature가 continuous space에서 emotional style을 잘 표현하는 tool이라고 생각하며, speaker-dependent emotion style transfer에 적합하다고 생각합니다.
이 논문의 main contribution은 다음과 같습니다. 1) 새로운 research topic인 expressive voice conversion을 정의합니다. 2) parallel data 없이도 학습 가능한 StarGAN 기반 expressive voice conversion framework를 제안하며, many-to-many conversion을 수행합니다. 3) publicly available multi-spekaer database를 이용해 실험을 진행하며, emotional style and speaker identity transfer에 있어 저자들이 제안한 method가 효과적임을 입증하였습니다. 저자들이 알기론 expressive voice conversion을 연구한 첫 논문입니다.
Speaker-Dependent Emotional Style
emotional feature extraction은 speech emotion recognition (SER)에서 매우 중요한 부분입니다. 많은 연구에서 deep neural network를 이용해 expressive speech에서 계층적 feature representation을 추출할 수 있다는 것을 보였습니다. 최근 speech synthesis 연구에서는 서로 다른 감정 style을 연속적인 공간에서 characterize 하는 deep emotional feature를 사용하였습니다.
저자들은 expressive voice conversion scenario에 대한 연구를 진행하며, source and target speaker가 각자 style대로 동일한 감정을 표현하고 있는 상태로 가정을 합니다. 이때 각자 style을 speaker-dependent emotional style이라 말합니다. emotion style은 계층적인 특성이 있기 때문에 명확하게 설명하기 어렵습니다. 저자들은 deep emotional feature가 speaker-dependent and speaker-independent emotional style 모두 포함하고 있다고 생각합니다. 그래서 저자들은 expressive voice conversion을 수행하기 위해 deep emotional feature를 사용합니다.
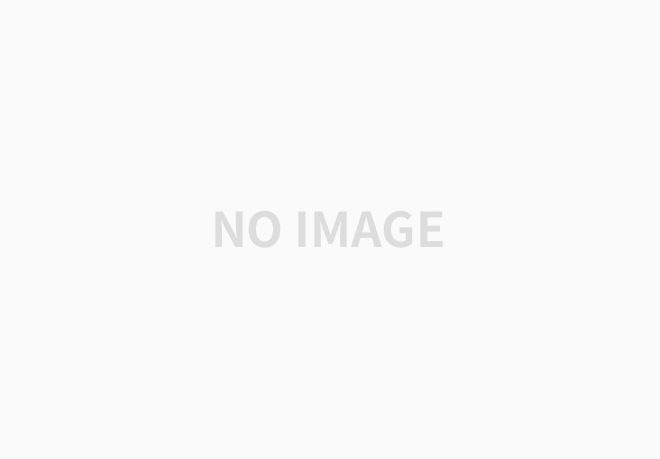
SER network를 학습한 후 last projection layer 이전의 emotional style feature를 사용합니다. 이를 t-SNE algorithm을 이용해 visualize 하면 다음과 같습니다.
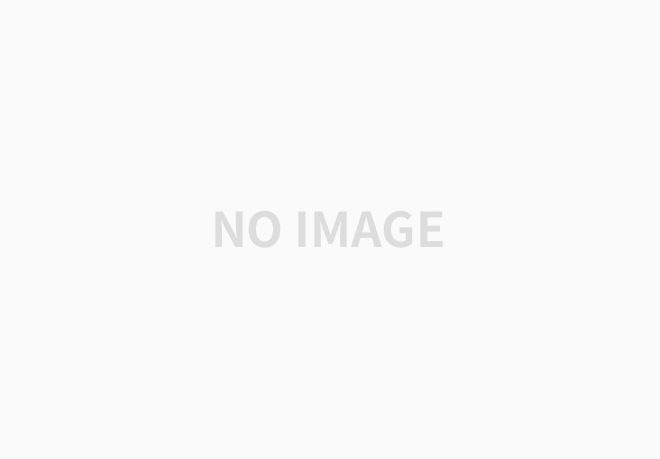
이때 2명의 여성(0016, 0018)과 남성(0013, 0020)을 사용했습니다. 위를 통해, 각 화자의 emotional style feature가 화자별로 emotion group을 형성하는 것을 볼 수 있습니다. 이를 통해 이 emotional style feature가 emotional state를 잘 characterize 하는 것으로 볼 수 있습니다.
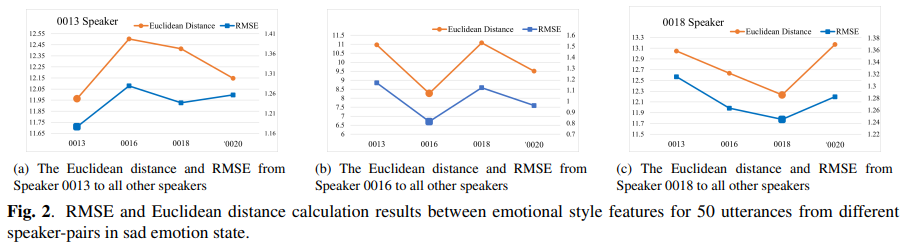
그리고 다른 화자에서 구한 다른 emotional style feature가 얼마나 다른 지 확인하기 위해, Euclidean Distance, RMSE를 사용하였습니다. 이를 통해 각 speaker pair마다의 emotional style feature 유사도를 측정했습니다. 위 결과를 통해, 동일 화자 내에서의 Distance와 RMSE가 다른 화자 간의 거리보다 작은 것을 볼 수 있습니다. 즉 SER에서 추출한 emotional style feature에는 speak-dependent information이 존재한다는 것을 의미합니다.
위 분석을 통해, emotional style feature를 사용한다면 speaker-dependent and speaker-independent emotional style을 사용할 수 있음을 보입니다.
A Joint Speaker Identity and Emtoion Style Transfer Framework
StarGAN을 사용하여 emotional style과 speaker identity을 동시에 변환하는 framework를 제안합니다. 이를 JES-StarGAN이라 부릅니다.
Stage Ⅰ: Emotional style descriptor training
emotional style은 speaker-dependent and speaker-independent characteristic을 동시에 표현하기 때문에, different emotion style을 one-hot emotion label과 같은 discrete representation을 사용하는 건 불충분합니다. 그러므로, 저자들은 large emotional speech corpus로 서로 다른 감정 style을 나타내도록 학습된 deep emotional feature를 사용합니다.
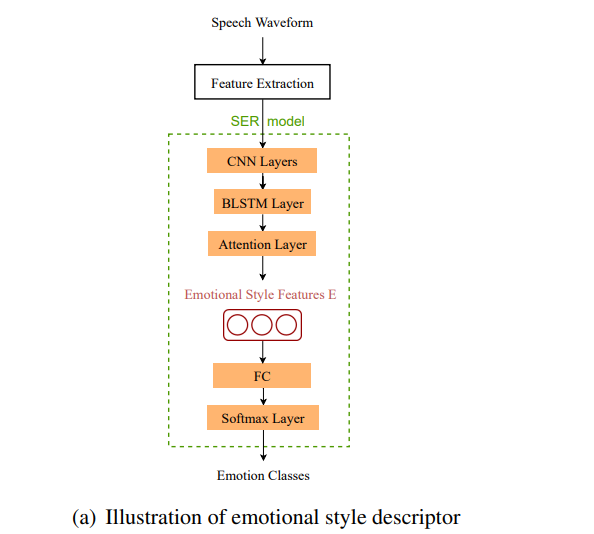
SER model이 다양한 화자의 다양한 감정으로부터 emotional style feature를 학습하도록 train 하였습니다. model architecture는 위 그림과 같습니다. SER network는 three-dimensional (3-D) CNN layer, BLSTM layer, attention layer, fully-connected (FC) layer로 구성됩니다. 먼저 3D CNN을 이용해 Mel-spectrum input을 고정된 크기의 latent representation으로 project 합니다. 이를 통해 감정에 관련 없는 element의 영향을 제거하면서 useful emotion information을 보존합니다. 그다음 BLSTM and attention layer가 temporal input을 summarize 하고 utterance-level feature를 생성합니다.
Stage Ⅱ: JES-StarGAN training
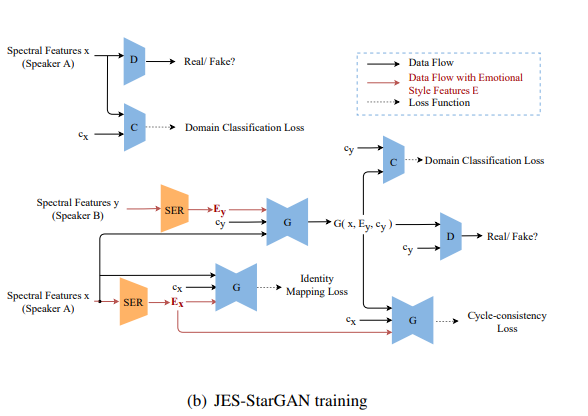
StarGAN은 parallel data 없이 many-to-many voice conversion을 수행하는 데 사용될 수 있습니다. 저자들은 StarGAN-based architecture를 제안하며, 구조는 위와 같습니다. generator G, discriminator D, domain classifier C로 구성됩니다. 저자들은 speaker identity와 speaker-dependent emotional style의 feature mapping을 학습합니다.
source acoustic feature sequence x, target speaker label cy, target emotional style feature Ey가 주어지면, generator는 emotional style feature Ey와 target speaker label cy를 condition으로 하여 source acoustic feature sequence x를 target domain으로 변환합니다. converted acoustic feature ˆy는 다음과 같이 작성할 수 있습니다.
ˆy=G(x,Ey,cy)
이 식에서 cy는 각 speaker를 나타내는 one-hot vector입니다. generator는 input feature로부터 speaker identity information과 speaker-dependent emotional style information을 동시에 학습합니다. discriminator D는 input이 real인지 아닌지 판별하도록 설계되어 있으며, classifier C는 input acoustic feature가 target speaker의 것인지 아닌지 구분합니다. 학습 과정은 위와 같습니다. training loss는 다음과 같습니다.
- Adversarial loss
G와 D를 학습할 때 사용되며 다음과 같습니다.

E[⋅]은 expectation을 의미합니다. cx은 source speaker의 speaker label, Ex는 source speaker의 emotional style feature를 나타냅니다. 학습하는 과정에서 D는 LDadv를 minimize 하고 G는 LGadv를 minimize 합니다. G의 경우, adversarial loss가 작을수록 converted speech와 target emotional speech 사이 더 높은 유사도를 나타내는 것을 의미합니다.
- Domain classification loss
domain classification loss를 이용해 C와 G를 학습하며, 다음과 같습니다.
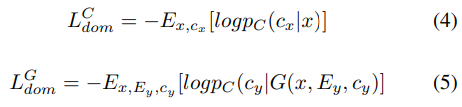
위 식에서 pC는 C가 구한 output probability distribution을 나타냅니다. 학습 과정에서 C는 real acoustic feature sequence x를 그에 맞는 speaker label cx로 분류하도록 학습되며, (4) 식을 최소화하면서 학습됩니다. G는 target domain cy로의 높은 분류 정확도를 보이는 acoustic feature sequence G(x,Ey,cy)를 생성하도록 학습됩니다. 이때 (5) 식을 최소화하면서 학습됩니다. G(x,Ey,cy)에서의 추가적인 input Ey는 G(x,Ey,cy)가 speaker-dependent emotional style information을 포함할 수 있도록 encourage 하여 C가 G(x,Ey,cy)를 target domain으로 더 쉽게 높은 정확도로 분류할 수 있도록 도와줍니다.
- Cycle-consistency loss
cycle-consistency loss는 input과 output의 contextual information이 일관되도록 보장하면서 speaker identity와 emotional style을 변환하도록 만들어 줍니다.

source speaker의 emotional style은 target speaker의 emotional style과 다르기 때문에, G(G(x,Ey,cy),Ex,cx)가 source acoustic feature와 더 가까워지도록 만들기 위해 G에 추가적인 condition으로 Ex를 input 하는 것은 필수적입니다.
- Identity mapping loss
동일한 source and target speaker가 사용될 때, linguistic information이 보존되도록 만들어줍니다.

speaker identity c와 Ex는 동일한 화자의 speaker-dependent feature를 나타냅니다. LGid는 G가 speaker-dependent feature를 고려하여 동일한 화자의 acoustic feature sequence가 일관성 있도록 만들어줍니다.
- full objective function
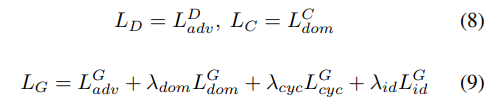
학습 과정에서 pre-trained SER model에서 얻은 emotional style encoding을 generator의 condition으로 사용합니다. JES-StarGAN은 generated acoustic feature의 distribution이 emotional data의 target feature distribution과 일치하도록 최적화됩니다. 추가적인 input emotional style feature를 사용해 JES-StarGAN은 emotional style과 speaker identity 모두 converted speech로 project 하는 것을 학습합니다.
Stage Ⅲ: Run-time conversion
run-time conversion 과정에서는 input utterance의 acoustic feature sequence x를 ˆy=G(x,E′y,cy)로 변환합니다. stage Ⅰ에서 구한 real target emotion style feature를 사용하지 않기 때문에, 저자들은 emotion style feature의 평균 E′y=mean(Erye)을 계산해 사용합니다. 이는 특정 감정 상태에 해당하는 target speaker의 emotion style feature의 평균을 나타냅니다.
Experiments
Experimental setup
5ms 마다 36-dim Mel-cepstral coefficients (MCEPs), WORLD vocoder를 이용해 fundamental frequency (F0), aperiodicity (APs)를 추출합니다. 36-dim MCEPs를 spectral feature로 사용하고 logarithm Gaussian (LG) normalized transformation을 통해 F0를 변환하고 source에서 APs를 추출해 사용했습니다.
SER network를 IEMOCAP dataset으로 학습한 후 ESD로 fine-tune 하였습니다. SER network를 통해 64-dim emotional style feature를 얻습니다. 그다음 full-connected layer를 이용해 emotion style feature, 36-dim MCEPs와 합쳐 generator의 input으로 사용합니다.
Objective evaluation
neutral, happy, sad utterance를 이용해 model 성능을 평가했습니다. converted and target speech 사이 Mel-cepstral distortion (MCD)를 계산했습니다. MCD 값이 낮다면 더 낮은 spectral distortion을 의미하며 더 나은 conversion performance를 보이는 것을 의미합니다.
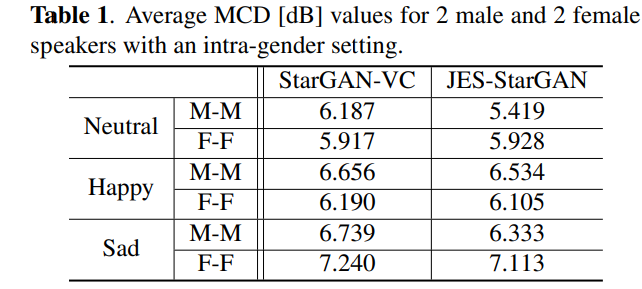
먼저 intra-gender에 대한 MCD result입니다. 저자들의 model이 더 좋은 성능을 보여줍니다.
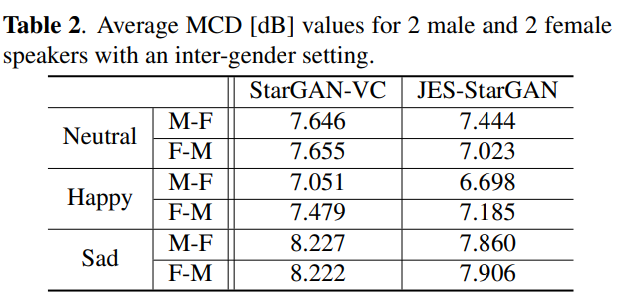
그 다음 inter-gender에 대한 MCD result입니다. 저자들의 model이 더 좋은 성능을 보여줍니다.
Conclusion
이 논문에서는 expressive voice conversion을 시도한 첫 연구입니다. 저자들은 expressive voice conversion problem을 정의하고 spekaer identity와 speaker-dependent emotional style을 동시에 변환할 수 있는 StarGAN 기반 solution을 제안합니다. 이는 parallel data가 필요하지 않습니다. 저자들은 continuous space에서 서로 다른 emotional style을 characterize 하기 위해 SER에서 deep emotional feature를 추출하여 사용했습니다. generator에 deep emotional feature를 condition으로 적용함으로써, 다른 화자의 speaker-dependent feature을 mapping 할 수 있도록 학습되었습니다. 실험을 통해 저자들의 model이 더 뛰어난 성능을 보이는 것을 입증하였습니다.